Biometric identity verification technology is at the forefront of screening, promising heightened security and an enhanced user experience. Among the latest advancements and digital identity verification trends we’ve covered in other blog posts, passive liveness detection has generated a lot of attention and the feature has been on the rise ever since.
It’s important to know the correct terminology. Understanding how the National Institute of Standards and Technology (NIST) categorizes liveness detection unlocks more insights into its purpose. The NIST classifies liveness detection as a subset of presentation attack detection (PAD) and defines it as follows: “The measurement and analysis of anatomical characteristics or involuntary or voluntary reactions, in order to determine if a biometric sample is being captured from a living subject present at the point of capture.”
Unlike traditional methods that rely on static biometric data, passive liveness introduces a dynamic layer of authentication to biometric identity verification to ensure the person behind the identity verification process is physically present and actively engaged. Applied to the use case of remote onboarding, it addresses the question, “Is the person taking the selfie really who they claim to be, or are they using a printed picture, mask, or other ‘spoofing’ technique to try and fool the IDV system?”
Let’s explore more in depth what passive liveness in biometric identity verification is and why it’s useful.
Understanding Passive Liveness Technology
A presentation attack is an attempt by an unauthorized individual to deceive a biometric authentication system using fraudulent means. This can include presenting fake biometric data or using spoofing techniques such as presenting photos, videos, or prosthetics that mimic biometric traits like facial features, fingerprints, iris patterns, or voice. By extension presentation attack detection (PAD) involves techniques and technologies designed to detect and mitigate these presentation attacks. PAD systems use various methods to verify the liveness of biometric traits being presented during authentication.
Early PAD “active liveness” technologies focused on instructing users to carry out voluntary reactions to prompts, such as tilting their head a certain way or following a randomly moving object with their eyes. These methods, while effective to a point, can be outsmarted with a little effort and ingenuity. Enter: passive liveness.
Technology vendors have come up with more advanced methods of detecting presentation attacks. These new methods fall under the category of “passive liveness”, as they don’t require the user to carry out any actions in order to allow the algorithm to calculate their liveness score. This is where things start to get interesting.
What’s Passive Liveness?
While active liveness technology is easy to understand, passive liveness in biometric identity verification is more of a mystery because it’s difficult to explain what’s actually happening in the background.
Passive liveness algorithms are neural networks trained using machine learning techniques on very large datasets containing many variations of spoof vectors (images of presentation attacks) such as printed masks, 3D masks, and images from mobile and PC screens. The training dataset also contains genuine selfie images and each image is labeled “genuine” or “fake.” The neural network then runs through multiple rounds of training, tweaking, and tuning until it’s able to detect presentation attacks with a high degree of accuracy using just one frame as a reference image.
Benefits of Passive Liveness
The good news is that research shows a significant increase in onboarding completion rates for organizations using passive liveness approaches:
- Active Liveness: 63% of customers successfully complete onboarding, taking an average of 13 seconds
- Passive Liveness: 99.9% of customers successfully complete onboarding, taking an average of 1 second
Another strong argument supporting passive liveness is the fact that there’s an ISO standard (30107-3), which sets out principles and methods for performance assessment of presentation attack detection mechanisms. A testing lab named iBeta, based in Denver, Colorado was the first to carry out testing according to this ISO standard in two levels; iBeta Level 1 PAD and iBeta Level 2 PAD.
Biometric benchmarks are useful to a point, however, we always recommend that you test biometric technology on your own data against two main criteria: speed and accuracy. If it’s very fast but not accurate, you can’t use it. If it’s very accurate but not fast, you shouldn’t use it either.
Enhanced Security and Fraud Prevention
The adoption of passive liveness in biometric identity verification enhances security measures. By detecting and preventing spoofing attempts with greater accuracy, passive liveness mitigates the risks associated with identity fraud, deepfakes, and other sophisticated impersonation techniques. This technology not only bolsters protection against fraudulent activities but also safeguards user data and maintains trust in digital interactions.
User Experience and Operational Efficiency
Beyond security benefits, passive liveness in biometric identity verification enhances user experience by eliminating the need for explicit user actions during verification. This seamless integration reduces friction in onboarding processes, enhancing user satisfaction and operational efficiency for businesses. Whether used in financial services, healthcare, or e-commerce, passive liveness technology ensures swift and secure identity verification without compromising convenience.
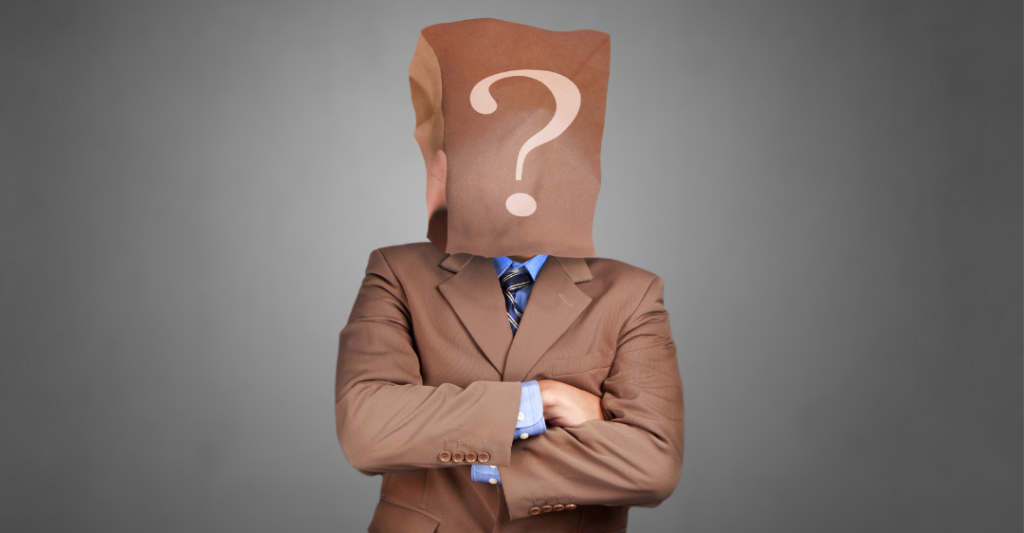
The Future of Biometric Identity Verification
In conclusion, passive liveness technology represents a transformative leap in biometric identity verification, combining improved security with enhanced user experience.
Passive liveness technology uses sophisticated algorithms to assess the liveliness of biometric features such as facial recognition or fingerprint scans. Unlike active liveness checks that require user interaction (like blinking or moving the head), passive liveness operates seamlessly in the background. It analyzes subtle behavioral cues, such as slight facial movements or changes in skin texture, to confirm the presence of a live person during the verification process.
As organizations across industries prioritize robust identity verification solutions, the demand for passive liveness technology is poised for rapid growth. Its role in combating evolving threats and enhancing digital trust positions it as a cornerstone of modern identity verification strategies. Looking ahead, advancements in AI and machine learning will further refine passive liveness capabilities, driving innovation and setting new benchmarks for secure digital identities.
By leveraging passive liveness to verify individuals without intrusive prompts, Trustmatic users can fortify their defences against fraud while delivering seamless digital interactions. As we navigate the complexities of digital identity, passive liveness emerges not only as an essential technology but also as a catalyst for trust and reliability in the digital age.
Talk to an expert via our identity verification service page to see how we can help.